The Nuances of AI Testing: Learnings from AI red-teaming
Artificial Intelligence (AI) Testing is a complex field that transcends the boundaries of traditional performance testing. While AI developers are well-versed with performance testing due to its prevalence in the educational system, it is crucial to understand that AI encompasses much more than just performance. In this post, I’d like to list some key principles in AI testing that are based on our experience at Validaitor while working with numerous partners and customers.
1. The trust deficit
A prevalent issue in the world of AI is the trust deficit between AI and its users. This lack of trust often results in underutilisation of AI. AI developers, in an attempt to bridge this gap, often lean towards obtaining external certification, even in the absence of regulatory purposes. And this is where independent AI testing adds most value.
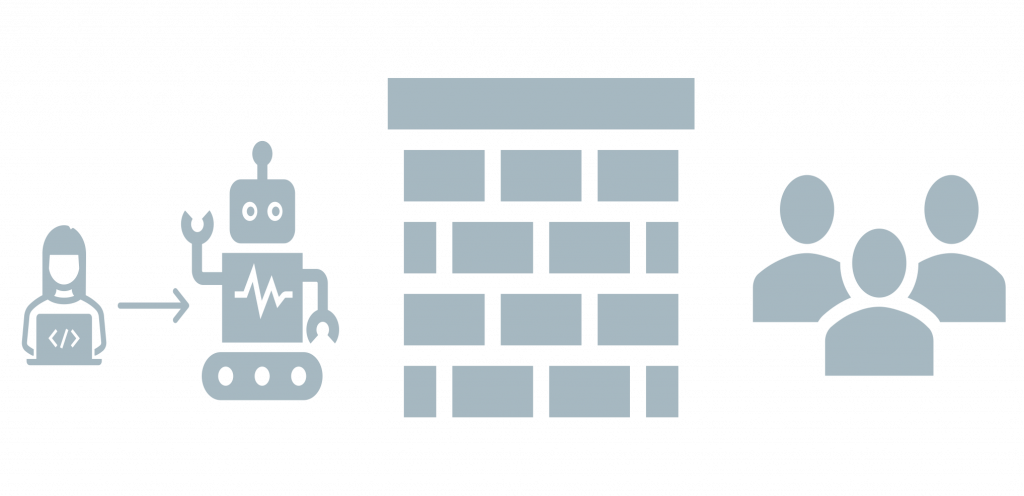
2. The benefit of AI testing
The overarching benefit of AI testing is to develop “better” AI. AI practitioners often anticipate actionable insights following AI testing. Identifying weak spots and generating suggestions for improvement should be the primary focus of any AI testing process. However, it’s important to remember that AI failure modes are often subtle and elusive. Especially with larger models, identifying weak spots becomes significantly more challenging.
3. Be ready for trade-offs
Creating robust AI often involves making a series of trade-offs. These include balancing security, fairness, robustness and privacy with performance. The task of achieving this balance is not an easy one, but it is a crucial step towards developing more trustworthy AI models.
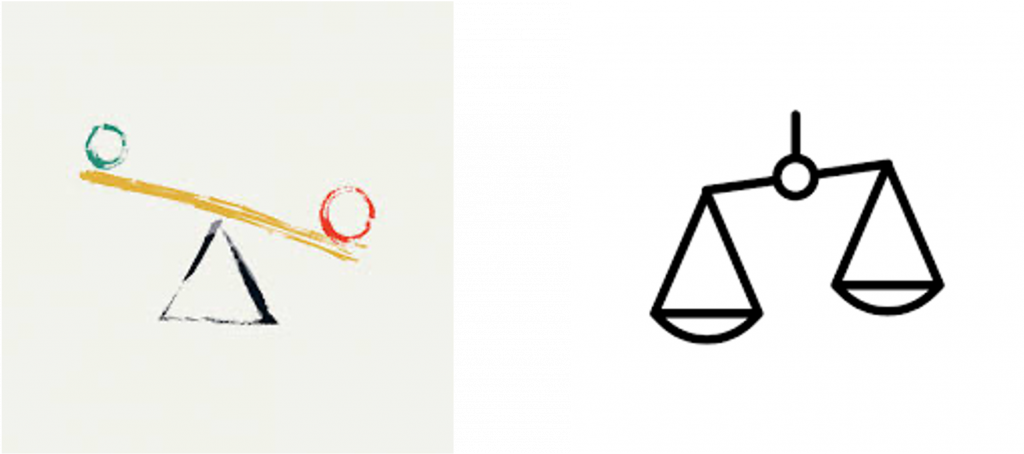
4. The hidden dangers of pre-trained models
The use of pre-trained models in AI development inadvertently spreads vulnerabilities. This issue is compounded by the current trend towards developing applications based on pre-trained large models like gpt4. The problems tend to cascade with each step of fine-tuning.
5. The challenge of testing general purpose models
Testing general-purpose AI models presents its own unique set of challenges. The larger the model, the harder it becomes to test and extract new insights. When a model is intended to be ‘general purpose’, defining a clear scope for testing becomes the only viable way forward.
6. Testing is use case specific
It’s also important to note that AI testing is use case specific. Tests only make sense if they’re justified within a specific use case scope. Different use cases will require different thresholds of acceptability.
7. Quantifiable metrics are key
AI testing also demands quantifiable metrics. Communication about the results of the testing process can only be effectively done using these metrics. Most often, the absence of metrics translates into no tests at all.
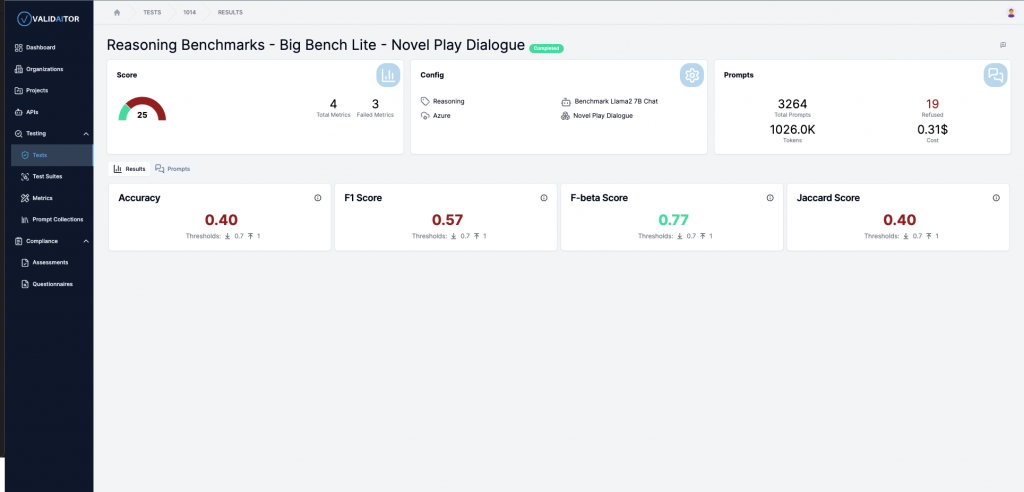
8. Transparency is the ultimate goal
Ultimately, the goal of testing in AI should be transparency. While it is virtually impossible to design a perfect test that uncovers all vulnerabilities, regulations should focus on enforcing transparency based on best effort. This will lead to a more robust, reliable, and effective AI systems that will be trusted by its users.
Conclusion
At Validaitor, we strive to be a bridge of trust between the AI developers and the society. In our journey so far, we’ve learnt a lot about the challenges of testing AI and how to overcome those challenges. We’re happy to share our learnings and insights with the community so that we can all work together to enhance AI safety and the responsible AI development.